DEEP LEARNING BASED AUTOMATED PNEUMONIA DETECTION AND CLASSIFICATION USING X-RAY IMAGES
DOI:
https://doi.org/10.71146/kjmr454Keywords:
Automated Pneumonia Detection, Chest X-Ray Imaging, Deep Learning Models, Support Vector Machine, Medical Image ClassificationAbstract
All over the world, pneumonia is a leading cause of sickness and death, so it’s critical to identify the condition soon and accurately to help patients. Reading chest X-ray films has long relied on the subjective judgments of radiologists, who may also need a lot of time. A method is proposed in this study to automatically find and classify pneumonia in chest X-ray images using deep learning and machine learning for the final diagnosis. The proposed strategy is designed using images of a publicly available chest X-ray pneumonia dataset with Normal or Pneumonia labels. At the beginning of preprocessing, noise must be removed, images must be normalized and more examples of data are generated to cope with class imbalance. An active contour method developed by Chan and Vese is introduced to segment lung regions and assist in obtaining accurate features. These networks automatically identify texture and shape details that illustrate important signs of pneumonia. Each machine learning classifier, Support Vector Machine (SVM), K-Nearest Neighbors (KNN) and Logistic Regression, is trained with these features and then tested on various groups of data. Results from the experiments prove that the SVM classifier is better than other models, as it delivered a test accuracy of 93.5%, a precision of 94.7%, a recall of 95.8%, and an ROC-AUC of 0.97. According to the results, the KNN method shows stronger test accuracy, while Logistic Regression still performs decently. The analysis also shows that SVM reduces the number of both false negatives and false positives, essential for making decisions in medical practice.
Downloads
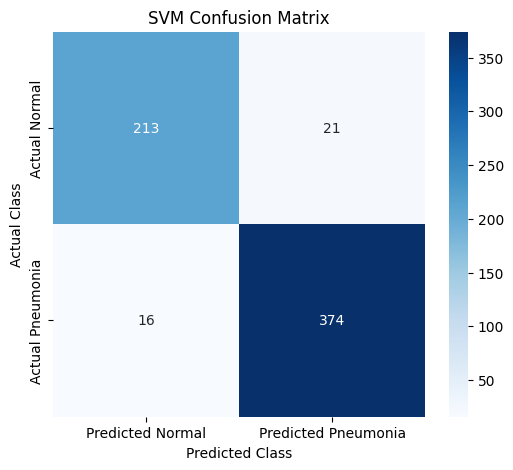
Downloads
Published
Issue
Section
License
Copyright (c) 2025 Muhammad Shabaz walee, Muhammad Kamran Abid, Moiz Ur Rahman, Sahrish Bashir, Muhammad Fuzail, Naeem Aslam (Author)

This work is licensed under a Creative Commons Attribution 4.0 International License.