SWARM INTELLIGENCE-BASED FEATURE SELECTION FOR SKIN CANCER DATA ANALYSIS USING CONVOLUTIONAL NEURAL NETWORKS
DOI:
https://doi.org/10.71146/kjmr283Keywords:
Skin Cancer, CNN, Swarm Intelligence, LSTM, RNNAbstract
In this paper, a comparative analysis of three widely used deep learning models to perform data classification tasks with and without a Swarm Intelligence (SI) based Feature Selection is presented. The study explores the performance of each model in the context of types of data it can handle and the effect of SI-based feature selection on its performance. Traditionally used for image-based tasks, CNNs outperformed RNNs and LSTMs with a baseline accuracy of 90%, capitalizing on their strength to learn spatial hierarchies in image data. The feasibility of SI for feature selection was demonstrated on RNNs in retrieving data time series (reaching 85% accuracy, which was 5% higher than a 80% baseline accuracy achieved without feature selection). Additionally, we investigated the generation and the execution of data collection, tasks which we encoded in the limited capability decision making module and subsequently plan to contextualize with appropriate application knowledge. SI based feature selection through Particle Swarm Optimization (PSO) has enhanced the performance of all model by selecting the most paneled features and brought the dimensionality of the input data on much lesser side. For CNNs we demonstrated a 4% gain in accuracy from 90% to 94%. In terms of RNNs and LSTMs the improvement was a bit smaller (but not by much), so that the accuracies rose from 80% to 85% for RNNs, and from 85% to 88% for LSTMs. The results demonstrate the significance of feature selection in deep learning models with high dimensional datasets because it not only improves accuracy but also decreases model deviation and computational expense. The study concludes generally that CNN models are better for image-based tasks while RNN models and LSTMs are better for sequential data. The integration of Swarm Intelligence-based feature selection worked out to be effective for all the models, promising the potential to increase the deep learning performance in practical applications.
Downloads
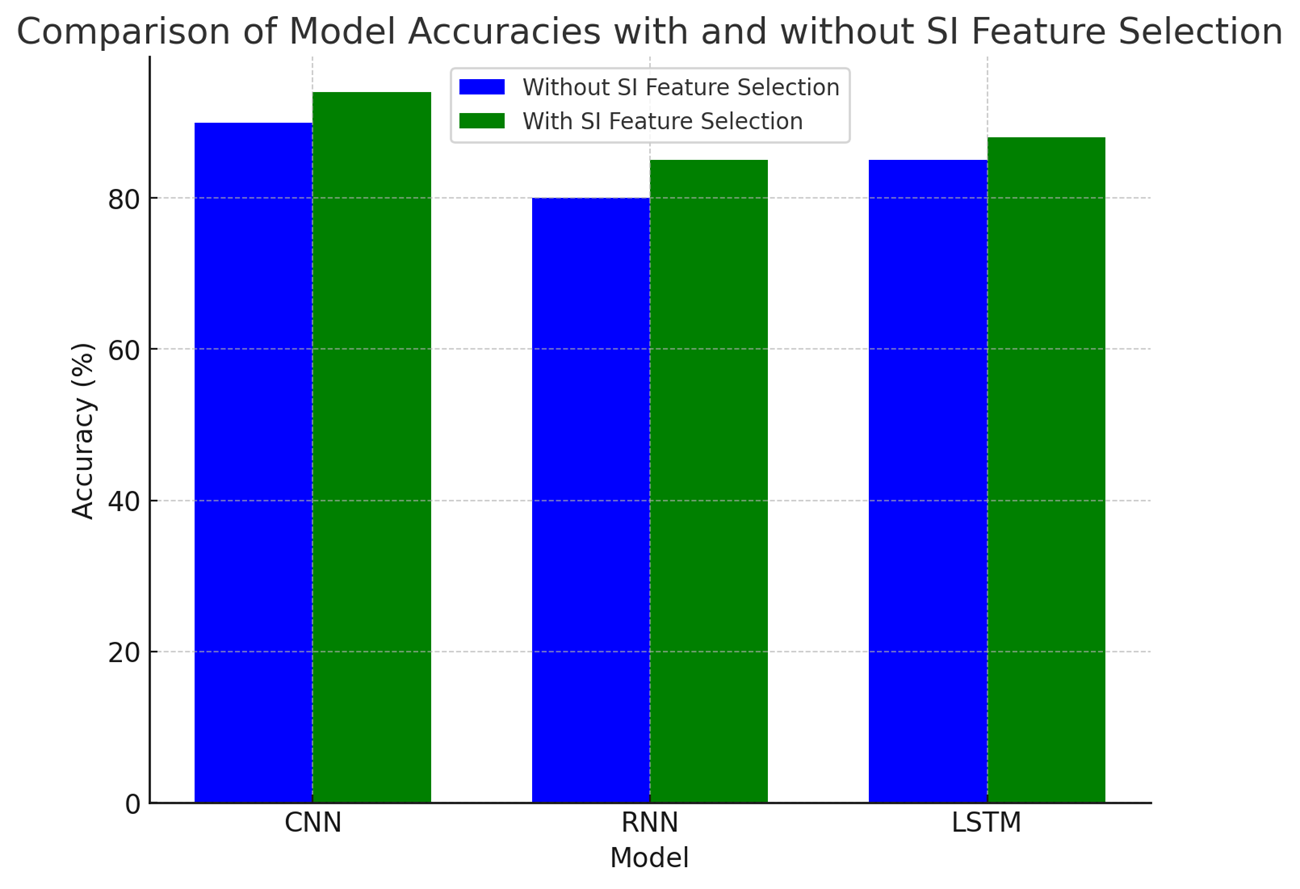
Downloads
Published
Issue
Section
License
Copyright (c) 2025 Kiran Manzoor, Muhammad Kamran Abid, Muhammad Fuzail, Yasir Aziz, Naeem Aslam, Nasir Umer (Author)

This work is licensed under a Creative Commons Attribution 4.0 International License.