HYBRID APPROACH FOR INTRUSION DETECTION USING MACHINE LEARNING
DOI:
https://doi.org/10.71146/kjmr331Keywords:
Intrusion Detection Systems, KNN, RF, SVM, Machine Learning (ML)Abstract
That is why the development of highly effective Intrusion Detection Systems IDS, protecting networks from both known and unfamiliar threats, has become especially actual due to the constant increase of the rate and complexity of cyber threats. The older approaches to IDS that are employed for classification based on signature and anomaly-based detection can sometimes prove themselves inadequate to deal with the emerging types of attacks. To overcome the above said limitations, this research puts forward a multiple machine learning classification technique of intrusion detection using a combination of three algorithms that is Support Vector Machine (SVM), Random Forest (RF) and K-nearest Neighbors (KNN). The proposed system therefore utilizes a combination of decision tree and K-NN algorithms with an intention of obtaining enhanced detection accuracy and decrements in false positives and false negatives in addition to generalization to a variety of attacking patterns. The methodology entails using stacking ensemble approach whereby three base classifiers namely SVM, RF and KNN are trained separately on network traffic data and the final result is produced by a meta-classifier. The effectiveness of the proposed hybrid model is established with the use of NSL-KDD dataset, a standard dataset in network intrusion detection. The findings further show that the proposed hybrid model outperforms the individual ML models in all the performance evaluation matrices of accuracy, precision, recall, and F1-score, indicating better generality and better appearance to identify the existing and new categories of attacks. Therefore, this research is useful in the domain of network security as it presents IDS using ensemble learning that is more deliberate in dealing with advanced. modern threats. Based on the results it is probable to conclude that the usage of the hybrid models is efficient for the real-time intrusion detection in the complicated networks.
Downloads
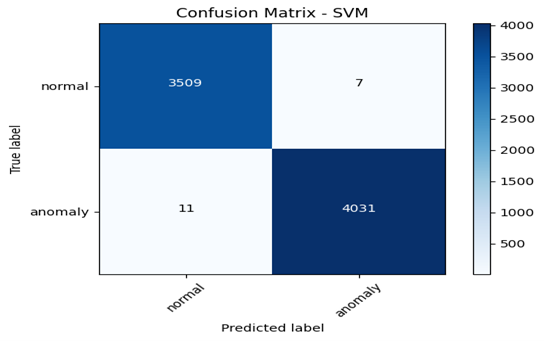
Downloads
Published
Issue
Section
License
Copyright (c) 2025 Muhammad Arslan Ayub, Ahmad Naeem, Muhammad Kamran Abid, Yasir Aziz, Naeem Aslam, Muhammad Fuzail (Author)

This work is licensed under a Creative Commons Attribution 4.0 International License.