UTILIZING DEEP LEARNING TECHNIQUES FOR DETECTING AND ANALYZING FOOD ALLERGIES
DOI:
https://doi.org/10.71146/kjmr332Keywords:
Deep Learning, Food Allergens, Hybrid Ensemble Model, Data NormalizationAbstract
There are promising trends in using deep learning approaches for identifying and studying food allergies which are increasingly becoming a major health concern in developed countries where millions of people suffer from some form of food allergy. Currently, ELISA and PCR are often used for the identification of food allergens, but both the methods are cumbersome and lack specificity and sensitivity, and therefore unsuitable for the largescale food allergen monitoring. This research aims at assessing the effectiveness of deep learning models such as CNN and RNN in enhancing the classification and identification of food allergens. Stellar such problems as data imbalance, the presence of low-quality datasets, and the interpretability of the generated models are solved by employing ensemble learning methods that can include Boosting and Bagging. These techniques take best characteristic from individual models to improve the prediction, and the hybrid models demonstrated higher ability in identifying allergens. Different techniques such as data cleaning and data normalization were applied on a data set obtained from Kaggle in order to build a better dataset for model building. The Hybrid Ensemble Model clearly yields higher accuracy, precision and F1 score than the other benchmark models like Logistic Regression and SVM. This paper reveals the viability of enumerating allergens using deep learning techniques with high accuracy, that can be implemented in industries and mark enhance consumer safety.
Downloads
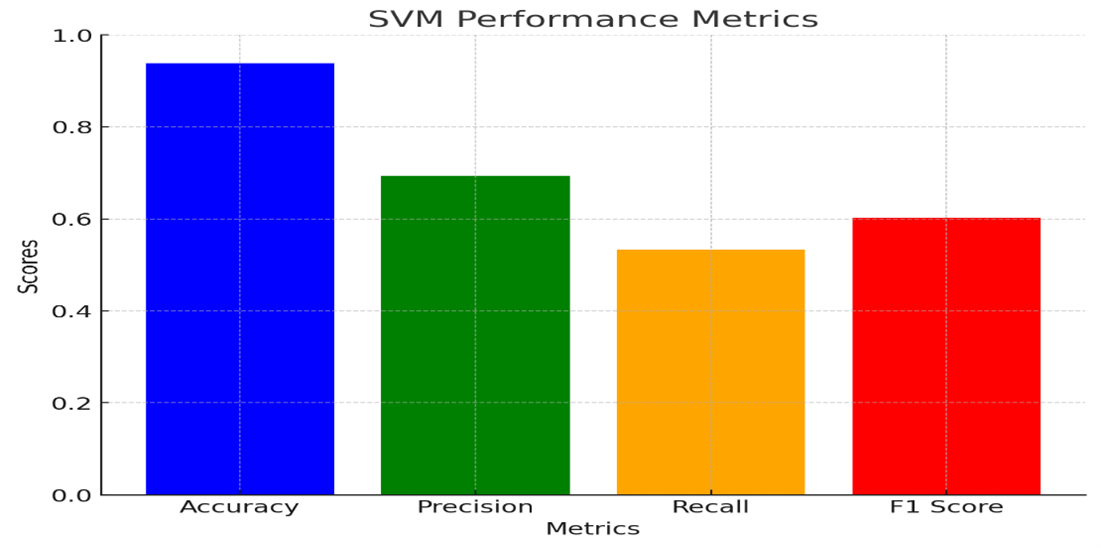
Downloads
Published
Issue
Section
License
Copyright (c) 2025 Junaid Ahmad, Naeem Aslam, Mian Abdul Qadeer Ahmad Khan, Muhammad Kamran Abid, Muhammad Fuzail, Nasir Umar, Talha Farooq Khan (Author)

This work is licensed under a Creative Commons Attribution 4.0 International License.