SYSTEMATIC REVIEW OF DEEP LEARNING TECHNIQUES IN EGG PLANT DISEASE DETECTION
DOI:
https://doi.org/10.71146/kjmr340Keywords:
Deep Learning, Hyper spectral data, Brinjal, Convolutional Neural Network (CNN) models and Image SensorAbstract
In recent years, researchers have focused on the automated identification of diseases using hyperspectral images, which is one of the most significant and basic difficulties for sustainable farming. The technology and approaches that have been employed up to this point are narrowly focused and entirely dependent on deep learning models. The most efficient technique for detecting and predicting illnesses from Brinjal pictures is convolutional neural networks. The current study examined several of the neural network processing methods already in use with the goal of identifying Brinjal illnesses. In order to handle the given imaging data, a number of deep learning models and architectures, image processing methods, and data gathering sources were first examined. After presenting the findings of the assessment of a number of currently used deep learning models, the study's conclusion included potential future applications of hyperspectral data processing. In order to identify diseases of the brinjal plant and facilitate further study into the wider possibilities of deep learning, this survey aims to enhance system performance and accuracy. Numerous image sensors and data collection devices were analyzed in order to identify plant illnesses. Lastly, we spoke about how deep learning models can do better than humans when it comes to generalization. In order to create an automated end-to-end plant disease management system, we conclude that realistic plant disease analysis will discover a number of crops, their related diseases early in the season, and correct disease severity assessment.
Downloads
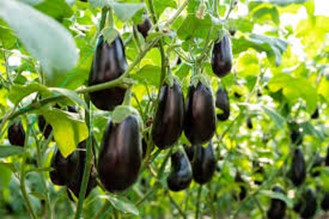
Downloads
Published
Issue
Section
License
Copyright (c) 2025 Saba Yousha, Nazish Iqbal, Abdul Kabeer Kazi, Hira Kalwar, Shahzad Nasim (Author)

This work is licensed under a Creative Commons Attribution 4.0 International License.