OPTIMIZED DEEP LEARNING FRAMEWORK FOR BRAIN TUMOR CLASSIFICATION USING GENETIC ALGORITHM-DRIVEN HYPERPARAMETER SELECTION
DOI:
https://doi.org/10.71146/kjmr404Keywords:
Brain tumor classification, Convolutional Neural Networks (CNNs), Genetic Algorithm (GA), Magnetic Resonance Imaging (MRI), Deep learning, Hyperparameter optimizationAbstract
Early and precise brain tumor detection is crucial to optimal treatment planning and patient outcome. Traditional MRI diagnosis tends to be time-consuming and subject to interobserver variations. For accurate and efficient diagnosis, we outline a deep learning framework with incorporation of Convolutional Neural Networks (CNN) coupled with Genetic Algorithm (GA)-assisted hyperparameter optimization for brain tumor automatic classification. The model is trained on a dataset of 7,023 MRI scans into Glioma, Meningioma, Pituitary, and No Tumor classes. For enhanced model robustness, Brain Data Augmentation (BDA) methods, such as affine transformation and intensity normalization, were performed. GA was used to tune important hyperparameters like learning rate, batch size, and epochs for training to enable better convergence and reduce overfitting. The VGG-16 model optimized showed a classification accuracy of 99%, with better sensitivity and specificity by tumor type. Confusion matrix analysis also proved that there was little misclassification, especially between tumors with similar morphology. This work highlights the potential of AI-based diagnostic platforms in neuro-oncology as a precise, reproducible, and efficient tool for the detection of brain tumors. Upcoming studies will investigate multi-modal image fusion, deep ensemble learning, and explainable AI methods for improving clinical relevance and decision support systems.
Downloads
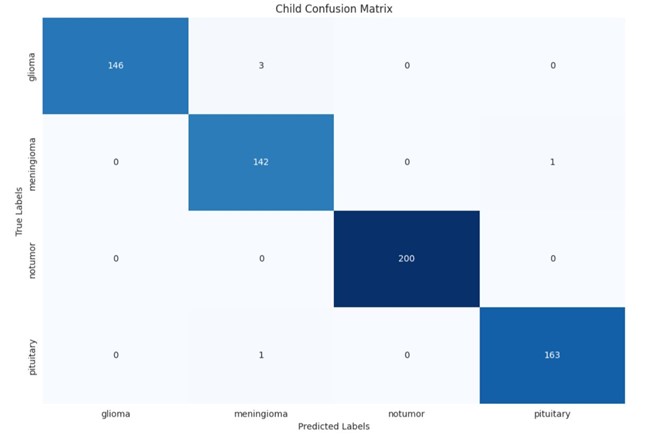
Downloads
Published
Issue
Section
License
Copyright (c) 2025 Muhammad Hamza Saleem, Ayesha Bibi, Naeem Aslam, Muhammad Fuzail, Mueed Ahmed Mirza, Hira Javaid (Author)

This work is licensed under a Creative Commons Attribution 4.0 International License.