IMPROVING THE EXPLAINABILITY AND TRANSPARENCY OF DEEP LEARNING MODELS IN INTRUSION DETECTION SYSTEMS
DOI:
https://doi.org/10.71146/kjmr284Keywords:
Intrusion Detection Systems, deep learning, LIME, SHAP, CNN, RNNAbstract
The conventional criteria of Intrusion Detection Systems (IDS) need to evolve because they fail to detect modern cyber security threats adequately. The advantages of machine learning (ML) and deep learning (DL) models in IDS functionality are limited by the inability to provide explanations which prevents cybersecurity professionals from validating decisions. The research analyzes DL-based IDS performance and interpretability standards through the examination of the NSL-KDD dataset. A screening process identified models that combined high reliability and accuracy numbers as selection candidates. feedforward neural networks (FNN), convolutional neural networks (CNN), and recurrent neural networks (RNN)—findings revealed that CNN achieved the greatest accuracy of 94.2% along with an AUC of 0.97 exempting FNN (91.3%) and RNN (93.8%). The effective extraction of spatial features from network traffic data by CNN models leads to its higher performance. The "black-box" nature of CNNs within DL models makes them difficult to understand because they remain concealed from users. The research integrated local interpretable model-agnostic explanations (LIME) and Shapley additive explanations (SHAP) to interpret decisions at the feature level. The implemented methods did not result in substantial accuracy improvements yet they made classification decisions more trustworthy and understandable by indicating the important features involved. Furthermore, in these developments there exist technical obstacles associated with high processing expenses and performance versus deployment speed balancing requirements. Research initiatives should focus on developing explainability techniques that maintain high-performance rates and excellent interpretability in IDS systems.
Downloads
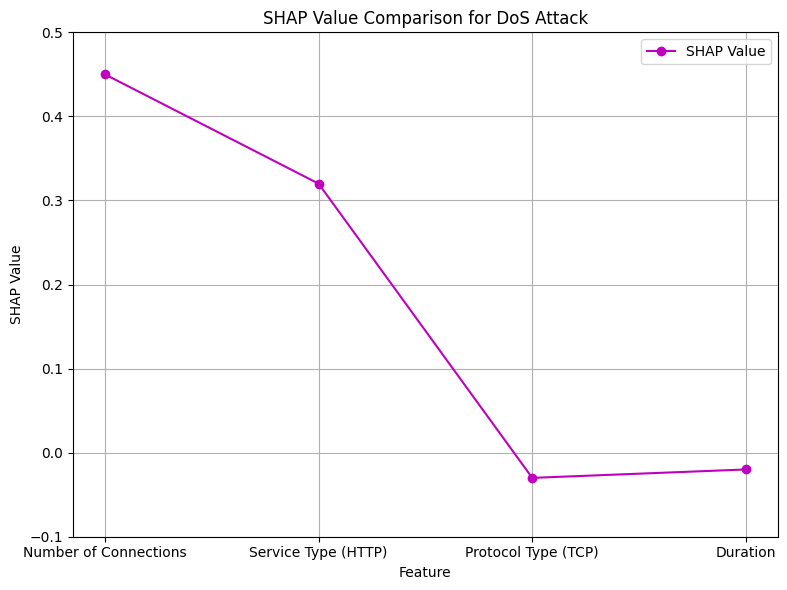
Downloads
Published
Issue
Section
License
Copyright (c) 2025 Daim Ali, Muhammad Kamran Abid, Muhammad Baqer, Yasir Aziz, Naeem Aslam, Nasir Umer (Author)

This work is licensed under a Creative Commons Attribution 4.0 International License.