TOWARDS SMART CATALYSIS: MACHINE LEARNING TECHNIQUES FOR ENHANCED PERFORMANCE IN DRY REFORMING OF METHANE
DOI:
https://doi.org/10.71146/kjmr230Keywords:
Dry Reforming of Methane (DRM), Catalyst Optimization, Machine Learning (ML), Neural Networks, Feature Importance Analysis, Sustainable Energy SolutionsAbstract
Catalysis supports today's chemical technology to create clean power while reducing harmful gases in our atmosphere. Dry Reforming of Methane (DRM) has gained attention as a valuable process to use methane and carbon dioxide for making synthesis gas which supports both clean energy production and chemical manufacturing. Traditional lab methods for improving DRM catalysts don't work well because they waste energy, make catalysts stop working faster, and fail to understand how multiple reaction elements relate to one another. The challenges of traditional methods drive researchers to use machine learning to find efficient solutions. Through our research, we present a system that combines machine learning models including regression analysis, decision trees, and neural networks to improve DRM catalyst development. Our neural network tests beat older methods by providing highly precise predictions with only a 3% deviation and an R² score of 0.98. As our analysis shows temperature stands out as the most important variable then time and catalyst makeup show secondary importance in helping guide catalyst development. Our method of using machine learning shortens the time needed to test and refine catalysts by 50%. Although this research offers useful outcomes the small data sample makes it hard to apply conclusions to other scenarios. Neural network operations remain unclear so scientists need SHAP analysis tools for better understanding. The next phase of the study should collect additional data and merge machine learning techniques with live experimental testing to discover superior catalysts and build practical heat-neutral DRM systems.
Downloads
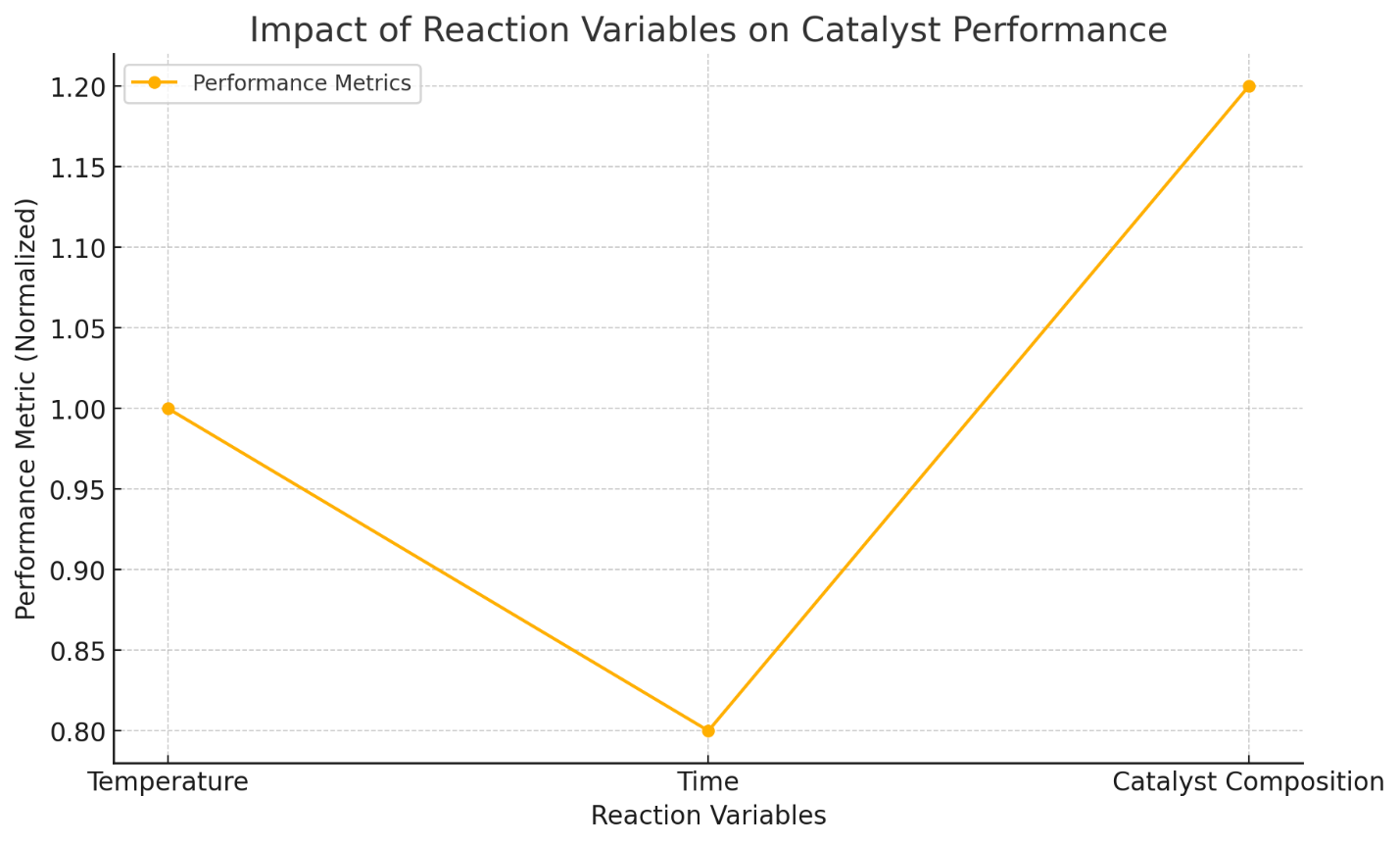
Downloads
Published
Issue
Section
License
Copyright (c) 2025 Subhan Azeem, Ayesha Bibi, Nadeem Hassan, Muhammad Kamran Abid (Author)

This work is licensed under a Creative Commons Attribution 4.0 International License.