PREDICTING COMPRESSIVE STRENGTH OF STEEL FIBERS REINFORCED CONCRETE INCORPORATING SILICA FUME USING MACHINE LEARNING MODELS
DOI:
https://doi.org/10.71146/kjmr186Keywords:
Steel Fibers Reinforced Concrete, Machine Learning, Artificial Neural Network, K-Nearest Neighbor, Random Forest, K-Fold Cross ValidationAbstract
Concrete is the most commonly used construction material around the world. As the compression strength of concrete goes up, the brittleness of the concrete matrix increases, hence terming it as a quasi-brittle material. This issue can be solved by the addition of steel fibers in the concrete mixture, which improve the post-peak load carrying mechanism of the concrete. Hence it becomes important to find the compressive strength of such improved concrete composites. In this study, a comprehensive dataset was developed by combining the findings from 17 research papers, which consisted of 8 participation variables, and compressive strength as the output variable. Three machine learning models were developed, i.e., Artificial Neural Network (ANN), K-Nearest Neighbors (KNN), and Random Forest (RF). It was observed that ANN model exhibited the highest value of R2 among the three models, i.e., R2=0.9941. To check the issue of overfitting in the best performing model, K-Fold Cross Validation technique was applied with five folds, which returned the average value of R2 as 0.9665, so validating the efficacy of the ANN model. In the last step, predictive equations were derived for the compressive strength calculation, as they eliminate the need for the model’s foundation file and hence enhance the significance of the research project.
Downloads
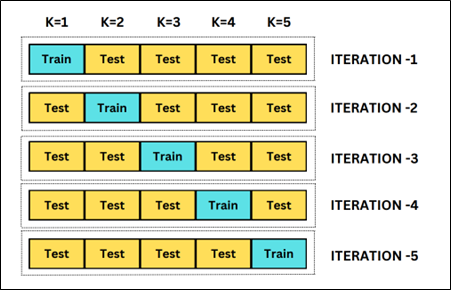
Downloads
Published
Issue
Section
License
Copyright (c) 2025 Aalia Faiz, Fakhar Imam, Shimza Jamil, Wasif Zubair, Ahmer Iqbal (Author)

This work is licensed under a Creative Commons Attribution 4.0 International License.