MULTI-DISEASE PREDICTION USING MACHINE LEARNING AND DEEP LEARNING MODELS
DOI:
https://doi.org/10.71146/kjmr176Keywords:
Classification, augmentation, feature selection, RandomForests, SVM Classifier, ANN, ResNet50, VGG16Abstract
In today's world, a large section of the human population suffers from treatable diseases such as heart problems, diabetes, skin cancer, stroke, liver disease, Parkinson's, malaria, and brain tumors. But due to lack of accessible and affordable healthcare the conditions may not be accurately or timely diagnosed that may lead to severe consequences, including disability or even death. Accuracy, affordability, timeliness and accessibility are critical factors that impact diagnosis quality. To improve diagnosis accuracy, affordability, accessibility and eliminate biases, machine learning and deep learning-based algorithms are gaining attraction in revolutionizing the healthcare industry. The objective of this study is to create a flexible and comprehensive medical diagnostic framework based on machine learning and deep learning models that can predict many diseases based on a patient's health records. The main objective of this study is to prevent problems caused by misdiagnosis and delayed diagnosis. By analyzing multiple diseases using a single platform, the cost of patient treatment can be reduced significantly, making it more accessible for people in underprivileged regions. By increasing the accuracy and speed of disease prediction, the proposed machine learning and deep learning-based diagnosis system has the potential to save lives. Experimental results showed that RandomForest has outperformed competing models on numerical datasets while on image datasets, VGG16 generated best accuracy than ResNet50.
Downloads
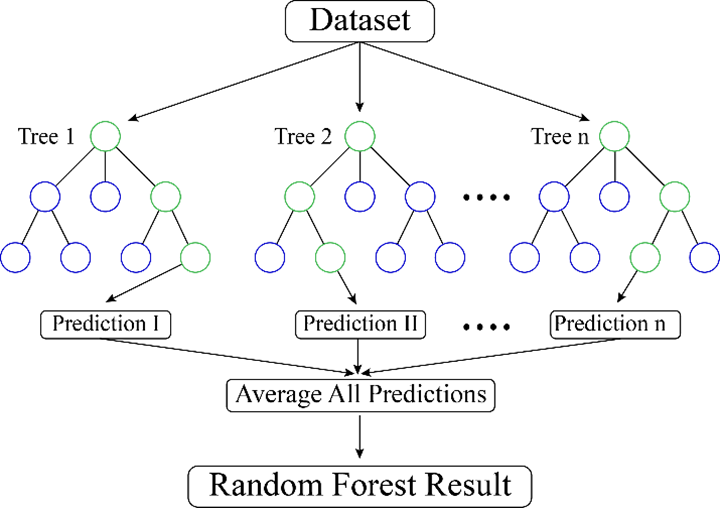
Downloads
Published
Issue
Section
License
Copyright (c) 2024 Fakhrud Din, Anwar Ul Haq, Muhammad Yaseen, Abdullah Khan, Asad Ali (Author)

This work is licensed under a Creative Commons Attribution 4.0 International License.