PREDICTING FLEXURE STRENGTH OF CERAMIC TILES MANUFACTURED BY INCORPORATING INDUSTRIAL WASTES AND COAL BOTTOM ASH USING MACHINE LEARNING MODELS
DOI:
https://doi.org/10.71146/kjmr199Keywords:
Ceramic Tiles, Machine Learning, Artificial Neural Network, K-Nearest Neighbor, Extreme Gradient Boosting, K-Fold Cross ValidationAbstract
The ceramic tiles industry is one of the largest industries globally and is expected to grow remarkably in the current decade. The ceramic tiles are a major source of decorations for building facades, interior design, etc. The manufacturing process of ceramic tiles is heavily dependent on the natural raw materials; hence a lot of research has been carried out to integrate various industrial wastes and coal ashes in its manufacturing. In this research project, an effort has been made to optimize the manufacturing process of ceramic tiles developed with the incorporation of industrial wastes and coal ashes, by predicting the flexure strength of ceramic tiles using different machine learning models. In this study, a comprehensive dataset was developed by combining the findings of 13 journal articles. The dataset consisted of 7 input variables, while the flexure strength of ceramic tiles was taken as an output variable. Three machine learning models were developed in this research project and their performance comparison was made. The developed machine learning models included artificial neural networks ANN, K-Nearest Neighbors and Extreme Gradient Boosting. In the later part of studies, K-Fold Cross Validation technique was applied to the best performing machine learning model to cross check the performance of the developed model. It was revealed that the ANN model performed the best among all the three machine learning models with an R2 value of 0.9795, which was authenticated by K-Fold cross Validation technique.
Downloads
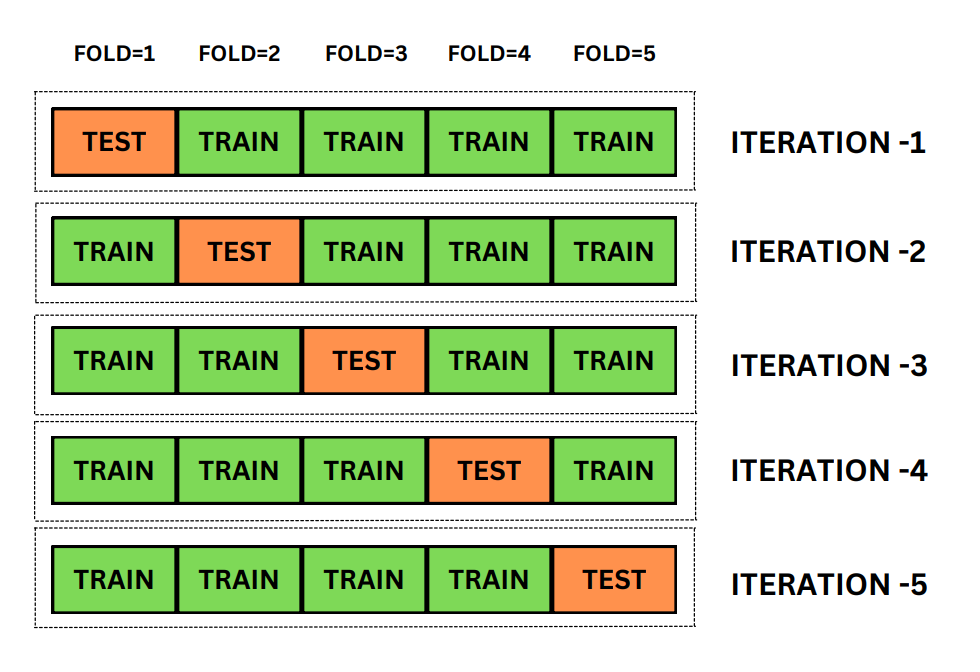
Downloads
Published
Issue
Section
License
Copyright (c) 2025 Aliyan Syed, Aalia Faiz, Shimza Jamil, Wasif Zubair, Fakhar Imam (Author)

This work is licensed under a Creative Commons Attribution 4.0 International License.