PREDICTING AND IDENTIFYING LAND FEATURES UTILISING REMOTE SENSING SATELLITE IMAGERY AND MACHINE LEARNING TECHNIQUES
DOI:
https://doi.org/10.71146/kjmr141Keywords:
Machine learning (ML), Convolutional Neural Networks, land cover and land use.Abstract
This study presents a comprehensive analysis of land feature identification in District Vehari, Pakistan, spanning from 1990 to 2025, utilizing remote sensing satellite imagery and machine learning (ML) techniques. The primary objective is to classify and analyze changes in land cover and land use over this period. The study employs a dataset consisting of multispectral satellite images from different years, along with corresponding ground truth data, for training and validation of ML models. Various ML algorithms, including Random Forest, Support Vector Machines, and Convolutional Neural Networks, are implemented and compared for their accuracy in classifying land features such as agriculture fields, water bodies, urban areas, and barren land. Preprocessing techniques such as image normalization, feature extraction, and dimensionality reduction are applied to enhance the performance of the models. The results reveal significant changes in land cover and land use patterns over the study period, with urban expansion and agricultural intensification being notable trends. The study concludes that ML techniques, when integrated with remote sensing data, provide an effective means for monitoring and analyzing land cover and land use changes in District Vehari. These findings are crucial for urban planning, agricultural management, and environmental conservation efforts in the region.
Downloads
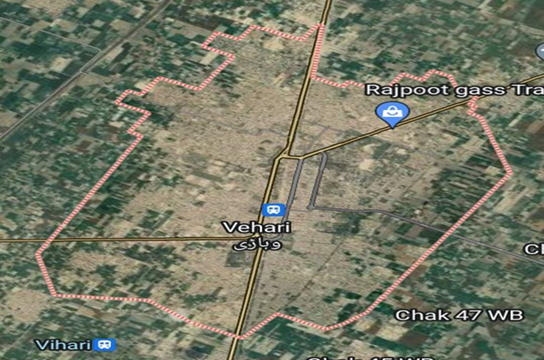
Downloads
Published
Issue
Section
License
Copyright (c) 2024 Sidra Saeed Rana, Iqra Irfan Rana, Dr. M. Jahangir Khan, Sidra Habib, Rana Muhammad Saleem, Hafiz M. Haroon, Huma Irfan (Author)

This work is licensed under a Creative Commons Attribution 4.0 International License.