DEEP CONVOLUTIONAL NETWORK FOR AUTOMATIC VIOLENCE DETECTION IN SURVEILLANCE VIDEOS USING TRANSFER LEARNING
DOI:
https://doi.org/10.71146/kjmr270Keywords:
Surveillance, redundant frame removal, Inception-v3, transfer learning, deep learning, violence detectionAbstract
Detections of violence within surveillance footage enable instant intervention support that advances public security measures. Traditional systems experience various implementation problems which include redundant frames as well as dependency on datasets and suboptimal generalization capacity. This paper presents an automatic violence detection system which applies Inception-v3 with transfer learning features to address existing system limitations. The system successfully performs effective and precise classification of video frames between violent and non-violent categories. The approach is a five-phase framework consisting of: (1) dataset collection (Hockey Fight and Real-Life Violence datasets), (2) elimination of redundant frames employing unsupervised learning and Euclidean distance-based similarity measures, (3) splitting of datasets (70% training, 30% testing), (4) transfer learning with Inception-v3, fine-tuned by substituting its last three layers, and (5) performance assessment. The system utilizes CNN-based feature extraction and the Stochastic Gradient Descent with Momentum (SGDM) optimizer for training. The proposed system delivers a performance rate of 73.86% and 78.61% on Hockey Fight and Real-Life Violence datasets surpassing other methods in state-of-the-art research. The proposed system improves processing speed and feature learning stability through redundant frame reduction techniques. The system demonstrates strong versatility among different situations which makes it suitable for use in real surveillance applications and content moderation systems. The proposed approach tackles problems with present approaches by developing a scalable solution for effective violence detection. The authors plan to focus on hyperparameter optimization and dataset testing as future work for accuracy enhancement.
Downloads
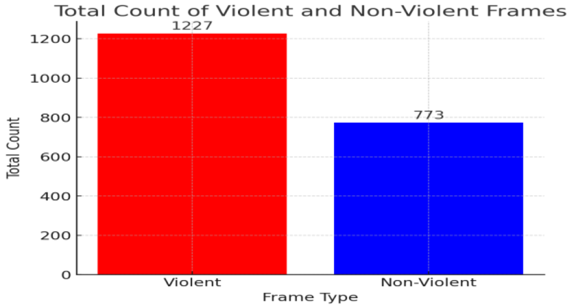
Downloads
Published
Issue
Section
License
Copyright (c) 2025 Muhammad Qasim Khan, Sohail Nawaz Sabir, Fazal Malik, Muhsin Khan (Author)

This work is licensed under a Creative Commons Attribution 4.0 International License.