DISEASE DETECTION IN PLANTS USING TRANSFER LEARNING APPROACHES
DOI:
https://doi.org/10.71146/kjmr218Keywords:
Image Classification, Transfer Learning, Convolutional Neural Networks (CNNs), Deep Learning, Pre-trained Models, Fine-tuning, Layer-freezing, Plant DiseasesAbstract
Food security completely relies on agriculture at the global level. Diseases of plants are a threat to crop yields which ultimately leads to economic loss and food insufficiency. With the boom of the technological revolution, traditional methods to detect diseases were left behind. Techniques from AI, computer vision, image processing, machine learning (ML), and deep learning (DL) were applied to automate processes and provide solutions quickly and labor-free. Transfer learning comes under the domain of machine learning, and can leverage pre-trained models on huge datasets, and acclimate them to certain tasks (detect plant disease). This research investigates the implications of the transfer learning (TL) approach in detecting plant diseases and getting better accuracy on larger datasets. The verdicts of this study have the potential to decrease the time and computational cost associated with model training and get more efficiency. This research used a dataset available on Kaggle named “plant disease recognition dataset”.
Downloads
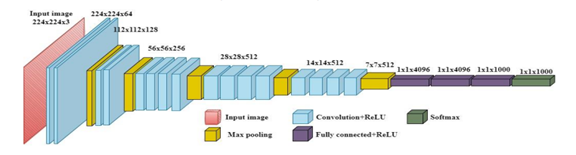
Downloads
Published
Issue
Section
License
Copyright (c) 2025 Warda Naeem, Shahid irfan, Zeeshan Ahmad, Shumaila iqbal, Muhammad Azam (Author)

This work is licensed under a Creative Commons Attribution 4.0 International License.