DETECTION OF DIABETES APPLYING MACHINE LEARNING TECHNIQUE
DOI:
https://doi.org/10.71146/kjmr125Keywords:
Support Vector Machine, Random Forest, Diabetics, Artificial IntelligenceAbstract
The health department can significantly benefit from adopting the latest advancements in technology, especially in the prediction and management of diseases. One of the most promising areas for improvement is the use of data-driven systems that leverage machine learning and artificial intelligence to analyze population health trends. By incorporating cutting-edge techniques, such as advanced predictive modeling and real-time data processing, the health department can enhance its ability to forecast public health outcomes, such as life expectancy. This would enable healthcare providers and policymakers to make more informed decisions, ultimately improving public health and reducing the burden on healthcare systems. The application of these techniques can be particularly useful in addressing chronic and life-threatening diseases, such as diabetes and cancer, which continue to pose significant challenges to global health. Diabetes and cancer, in particular, are among the leading causes of death worldwide. Despite the extensive research on these diseases, the global mortality rate continues to rise, highlighting the need for more targeted approaches. While numerous tools and methodologies have been developed to predict the spread of these diseases, there remains a crucial research gap: the lack of investigation into the attributes that directly contribute to the development of diabetes. Most existing models focus on the prediction of disease outcomes but fail to delve deeper into identifying the underlying causes, such as genetic factors, lifestyle choices, or environmental influences. This gap in research suggests a pressing need for the development of a system that not only works quickly but also operates with high accuracy in identifying the key attributes responsible for diabetes. By addressing this gap, it is possible to design interventions that are more effective in prevention and treatment, leading to better long-term health outcomes for populations at risk.
Downloads
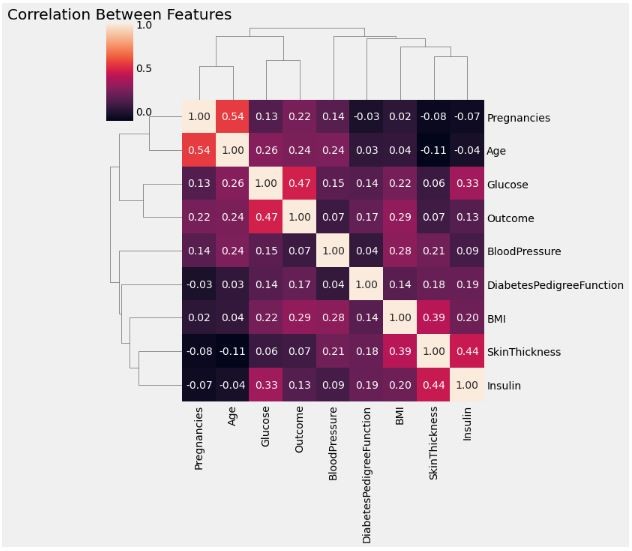
Downloads
Published
Issue
Section
License
Copyright (c) 2024 Nadia Shoukat, Salahuddin, Meiraj Aslam , Inzamam Shahzad (Author)

This work is licensed under a Creative Commons Attribution 4.0 International License.