WORKLOAD CHARACTERIZATION AND ADAPTIVE RESOURCE ALLOCATION IN EDGE-CLOUD ENVIRONMENTS USING BI-DIRECTIONAL LSTM NETWORKS
Keywords:
characterization, Resource allocation, Operating systems design, Heterogeneous systems, Distributed computing, Edge computing, Cloud computing, Machine learning, Bi-Directional LSTMAbstract
Resource management is crucial for performance and energy consumption of running operating systems especially in the heterogeneous distributed infrastructure of edge and cloud computing. This paper focuses on the methodological contributions (2020–2024) cataloged in the literature toward workload characterization, emphasizing the importance of task behavior and resource variability. We propose an advanced facilitating tool called the ‘Obligation Profiler’ which links Obligation Voting with statistical profiles and Bi-Directional Long Short-Term Memory (LSTM) neural networks to map workflow intricacy. BD LSTM architecture provides more accurate identification of workloads by making use of the forward and backward information of the data. Using this model, we make reasonable and adaptive assignment decisions for allocating computational resources across the tasks. The current framework is tested in several computing platforms and shown to generate enhancements on the system parameters, power consumption, and scalability. The principal lesson from this work is that there is a need to integrate the use of different machine learning techniques like LSTM with more traditional resource management approaches.
Downloads
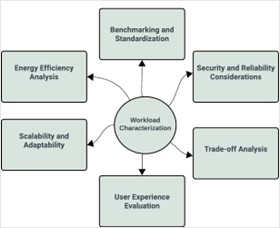
Downloads
Published
Issue
Section
License
Copyright (c) 2024 Zunaira Rashid, Mujeeb Ur Rehman, Akbar Hussain, Imtiaz Hussain

This work is licensed under a Creative Commons Attribution 4.0 International License.