Subcellular Structures Classification in Fluorescence Microscopic Images
Keywords:
Fractal Texture Analysis, Support Vector Machine, local binary patternAbstract
Evaluation of cellular protein localization is becoming progressively important. Even after the whole human genome has been sequenced, numerous years will be required to study the structure, function, and each protein localization. Localization information is very important because it provides a context for a protein’s structural and functional information. For instance, two proteins that possess comparatively similar function and structure may in fact be found in different compartments within the cell hence, these might be included in unrelated cellular processes. Localization data for several proteins were collected using fluorescence microscopy. The resulting patterns were described using a variety of numeric features including local binary pattern (LBP), Haralick’s texture features, edge histogram descriptor (EHD), Segmentation-based Fractal Texture Analysis (SFTA) and after that these features were combined to form a single feature vector. This feature vector is then given as input to the classifier to classify the image into 10 different classes for 2D Hela dataset and into 5 classes for CHO dataset. Support vector machine (SVM) with chi-square kernel is used to solve the classification problem. Proposed frame-work obtained 98.9% overall accuracy on Hela dataset and 99.6% on CHO dataset. The obtained accuracy is 0.9% higher on 2D Hela dataset and 0.6% higher on CHO dataset as compared to highest computed accuracy.
Downloads
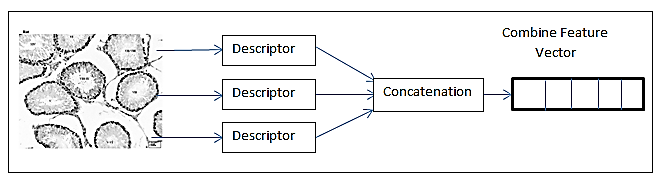
Downloads
Published
Issue
Section
License
Copyright (c) 2024 Saima Batool , Ghazanfar Ali, Inzamam Shahzad, Muhammad Hanif Soomro, Salahuddin, Hafiz Muhammad Ijaz (Author)

This work is licensed under a Creative Commons Attribution 4.0 International License.